Why Transparent Labeling of Training Data is Essential for Building Trust in AI: Expert Insights
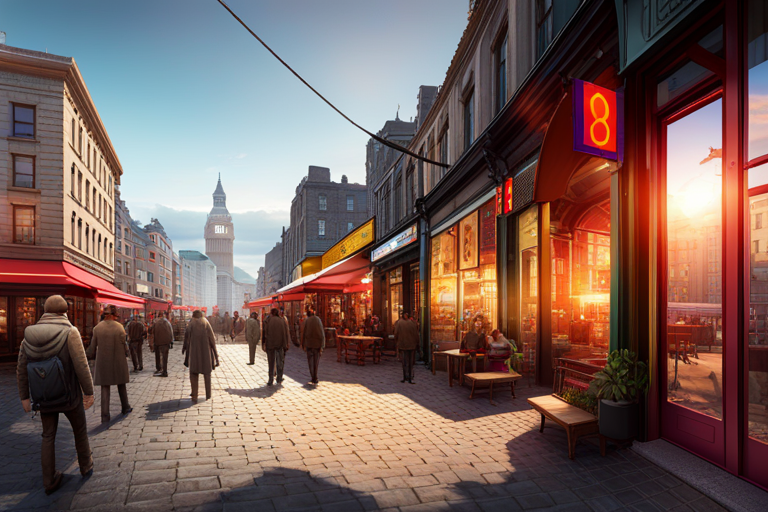
Why Transparent Labeling of Training Data is Key to Building Trust in AI
As the chief editor of mindburst.ai, I've seen the rapid growth of artificial intelligence in recent years. While exciting, AI has also been met with skepticism and even fear due to concerns around transparency and accountability. That's why I believe transparent labeling of training data is crucial to building trust in AI. Here's why:
The problem with opaque training data
When AI is used to make decisions that impact people's lives - from hiring decisions to criminal justice - it's essential to understand how the machine arrived at its conclusion. However, opaque training data, where the data used to train the AI is not disclosed, makes this impossible. This lack of transparency can lead to mistrust and suspicion of AI, which can undermine its potential to make a positive impact.
The benefits of transparent labeling
On the other hand, transparent labeling of training data can help build trust in AI by providing a clear understanding of how the machine arrived at its decision. This not only increases transparency but also allows for easier identification of bias and other issues that may need to be addressed.
Examples of transparent labeling in action
Here are a few examples of how transparent labeling is being used in the real world:
- Google's AI Explainability whitepaper outlines their approach to transparent labeling, which includes providing a clear explanation of how their AI systems make decisions.
- IBM's AI Fairness 360 toolkit includes tools for identifying and mitigating bias in AI systems, which can help build trust with users.
- The European Union's General Data Protection Regulation (GDPR) requires organizations to disclose the use of AI in decision-making and to provide an explanation of how the machine arrived at its decision.
The future of transparent labeling
As AI continues to become more integrated into our daily lives, transparent labeling will become increasingly important. I believe that in the future, we'll see more regulations and standards around transparent labeling, and AI companies will need to prioritize transparency to build trust with users.
In conclusion, transparent labeling of training data is key to building trust in AI. By providing a clear understanding of how AI systems make decisions, we can increase transparency, identify bias, and ultimately ensure that AI is used in a fair and ethical way.