Rethinking AI Benchmarks: Why We Need a More Comprehensive Approach
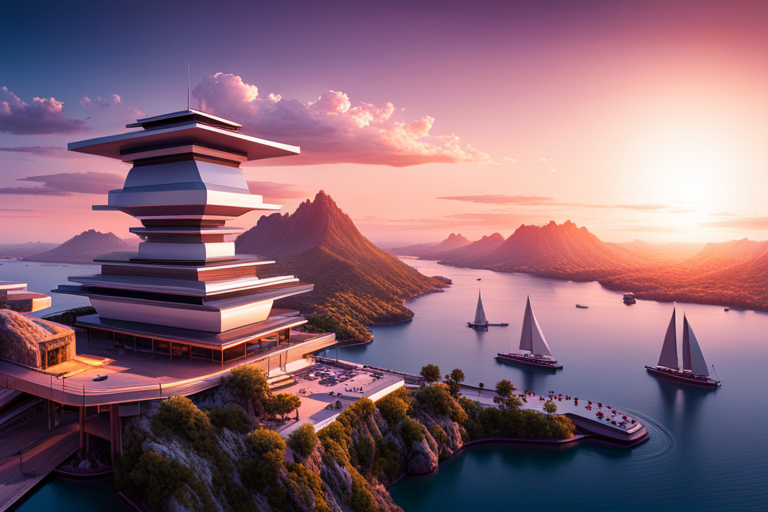
Rethinking AI benchmarks: Why we need to challenge the status quo
As the chief editor of mindburst.ai, I am always on the lookout for the latest developments in artificial intelligence. Recently, a new paper has caught my attention, challenging the way we evaluate AI benchmarks. This is a crucial issue that we need to address, so let's dive into the details.
The problem with traditional AI benchmarks
Traditional AI benchmarks have been used for many years to evaluate the performance of AI models. These benchmarks are designed to test the accuracy, speed, and efficiency of the models, using standardized datasets and metrics.
While these benchmarks have been useful in the past, they are no longer sufficient for evaluating modern AI models. Today's AI models are more complex and diverse, and they require a more comprehensive evaluation approach.
The limitations of current AI benchmarks
The problem with current AI benchmarks is that they are often based on narrow, specific tasks, such as speech recognition or image classification. This means that they can be easily optimized for these tasks, without necessarily improving the overall performance of the AI model.
Another limitation of current benchmarks is that they often focus on a single metric, such as accuracy or speed. This can lead to models that are optimized for a specific metric, at the expense of other important factors, such as interpretability or fairness.
A new approach to AI benchmarking
The new paper proposes a more comprehensive approach to AI benchmarking, which takes into account a wide range of factors, including:
- Accuracy: how well the model performs on a given task
- Robustness: how well the model performs on tasks outside of its training data
- Interpretability: how easy it is to understand how the model makes its decisions
- Fairness: how well the model avoids bias and discrimination
By taking all of these factors into account, we can create a more holistic evaluation of AI models, which will help us to identify their strengths and weaknesses more accurately.
The future of AI benchmarking
As AI continues to evolve and become more complex, we need to rethink our approach to benchmarking. We need to move away from narrow, task-specific benchmarks and towards a more comprehensive evaluation approach.
This will require a collaborative effort from researchers, developers, and industry experts. We need to work together to create new benchmarks that reflect the complexity and diversity of modern AI models.
In conclusion, the traditional approach to AI benchmarking is no longer sufficient for evaluating modern AI models. We need to challenge the status quo and adopt a more comprehensive approach that takes into account a wide range of factors. By doing so, we can ensure that AI continues to develop in a way that is accurate, robust, interpretable, and fair.