Protecting AI: Understanding Data Poisoning Threats
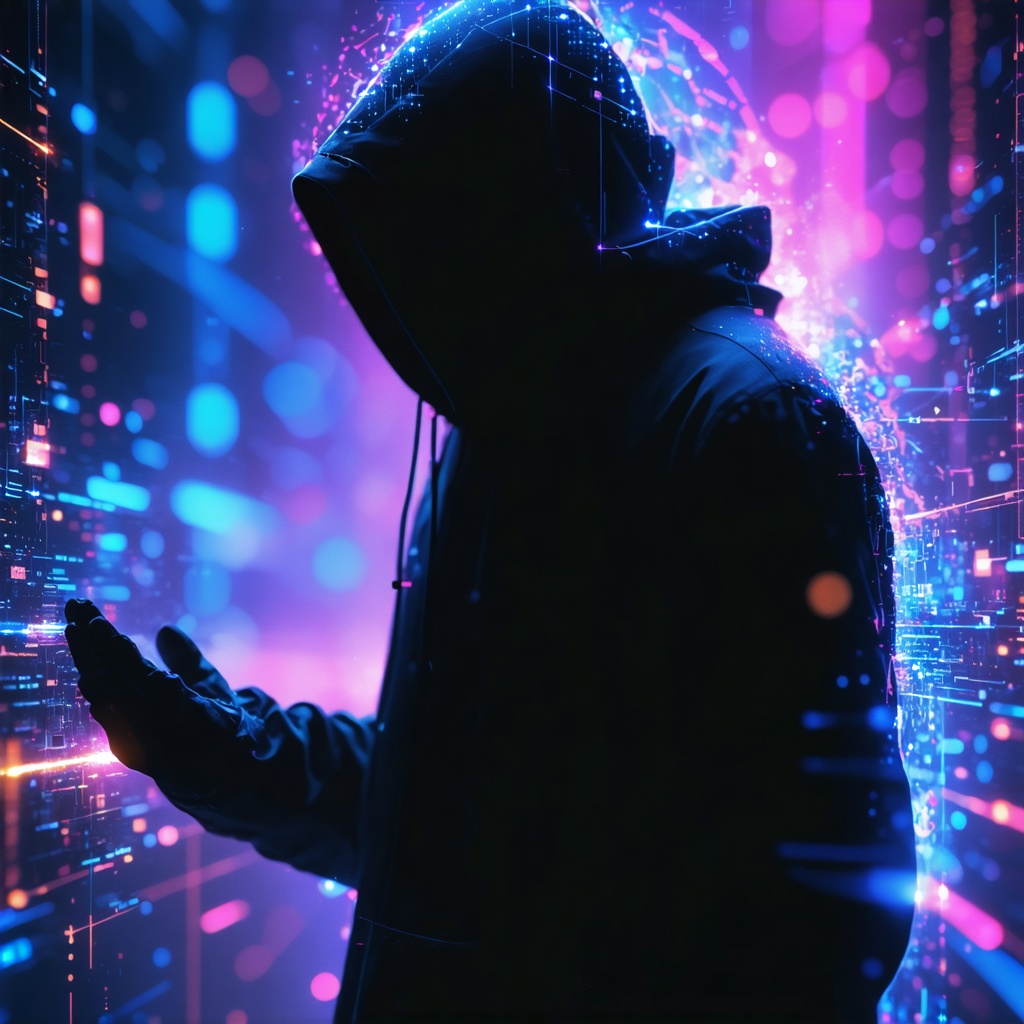
Data Poisoning: The Sneaky Villain in the AI World You Need to Know About!
As the chief editor at MindBurst.ai, I've seen my fair share of cyber threats, but data poisoning has emerged as one of the most insidious and cunning. Imagine someone slipping a few rotten apples into a basket of fresh ones, and suddenly, the entire batch is compromised. That's data poisoning for you—a subtle yet powerful attack that can undermine even the most sophisticated AI systems. Let’s dive into the three critical aspects of this emerging threat that everyone in the tech community should be aware of!
1. What is Data Poisoning, Anyway?
Data poisoning occurs when an adversary manipulates the data used to train machine learning models. This can lead to:
- Inaccurate Predictions: The model learns from tainted data, resulting in flawed outcomes.
- Model Malfunction: Some attacks can cause the model to behave erratically.
- Trust Erosion: Users may lose faith in AI systems due to compromised outputs.
Why Should You Care?
As AI systems permeate industries from finance to healthcare, the stakes are higher than ever. A single successful poisoning attack could result in financial losses, data leaks, or even safety hazards, depending on the application. For more insights on the potential risks of AI, check out MoneyGPT: AI and the Threat to the Global Economy.
2. The Methods: How Do Attackers Pull This Off?
There are various techniques that attackers can employ for data poisoning, and understanding them is crucial for defense. Here are a few common strategies:
- Label Flipping: Changing the labels of training data points to mislead the model.
- Backdoor Attacks: Inserting malicious data that triggers a specific response only when certain conditions are met.
- Data Injection: Introducing entirely new, corrupted data points into the training set.
The Takeaway
Attackers are getting more creative, and the more we know about their tactics, the better we can defend against them. If you're looking to dive deeper into adversarial tactics, consider reading Adversarial AI Attacks, Mitigations, and Defense Strategies.
3. The Defense: What Can You Do?
With great power comes great responsibility, and that rings true for AI developers and users. Here are some strategies to protect your models:
- Robust Data Validation: Implement rigorous checks to ensure data quality before it enters your training set.
- Adversarial Training: Expose your models to potential attacks during training to make them resistant.
- Regular Monitoring: Continuously evaluate model performance and behaviors to catch anomalies early.
Your AI's Best Defense
Remember, prevention is always better than cure. By being proactive about these defenses, you can significantly mitigate the risks associated with data poisoning. For comprehensive guidance on protecting AI from cyber threats, check out Secure Intelligent Machines: Protecting AI from Cyberattack.
Wrapping It Up: Stay Vigilant!
Data poisoning may not grab headlines like ransomware or DDoS attacks, but it’s a threat that lurks in the shadows, waiting for the opportune moment to strike. As AI continues to evolve, so too must our understanding and defenses against these hidden dangers. Stay informed, stay cautious, and let’s keep our AI systems healthy and trustworthy! For a broader perspective on the implications of AI, consider exploring Uncontrollable: The Threat of Artificial Superintelligence and the Race to Save the World.